Which content influenced the MQLs created in a time period?
How to use Dreamdata to measure which content affected the MQLs created in a selected time period
In Dreamdata, the pipeline stages are defined using the Stage option.
First step is to choose which stage to analyse. Below we use Marketing Qualified Lead (MQL) as an example.
To analyze which content the influenced MQLs generated in a selected time period, we set the dashboard options as shown.
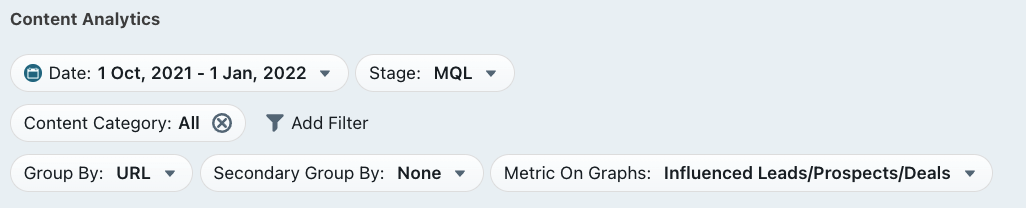
This will setup the report to investigate:
- Which content influenced the MQL's generated between 1 Oct 2021 - 1 Jan 2022?
To consider what pipeline was generated by content views in a certain time period, go to Content Performance.
The Group By option indicates the granularity of the content we want to show. if we are tracking a substantial amount of individual url’s, it might provide a clearer view to aggregate them by content category. Note that content categories need to be setup within the Dreamdata application (see here)
Here we leave The Secondary Group By to None as we do not want to further split the URL in this analysis.
The top part of the dashboard shows the total number of selected Leads on the left and on the right it shows the number of those Leads that was influenced by some content.

The graph shows the top 10 urls that influenced the most of the total selected MQLs.
This can be used to quickly identify the content that influenced the most MQLs generated in the selected period.
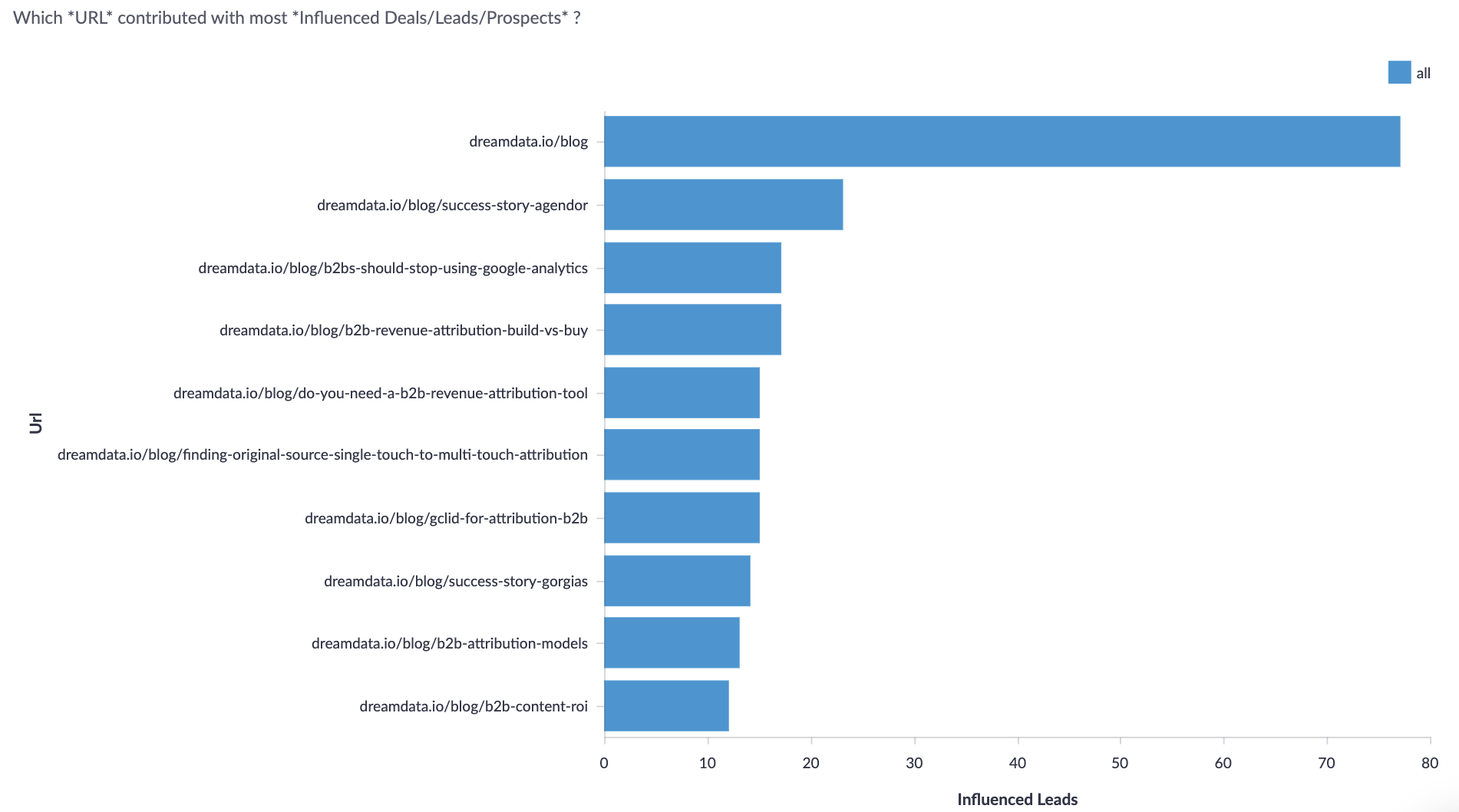
The summary table shows detailed information for each url or category. Note that no secondary group by is selected in this analysis, so it is showing all (= no split groups).
The first table shows the number of contacts from the MQLs that read each content piece.
The table is ordered by the number of Influenced Leads (and the percentage of selected Leads influenced), shows which content affected the most of the MQLs thereby identifying the content that was consumed by readers that became MQL in the selected time period.
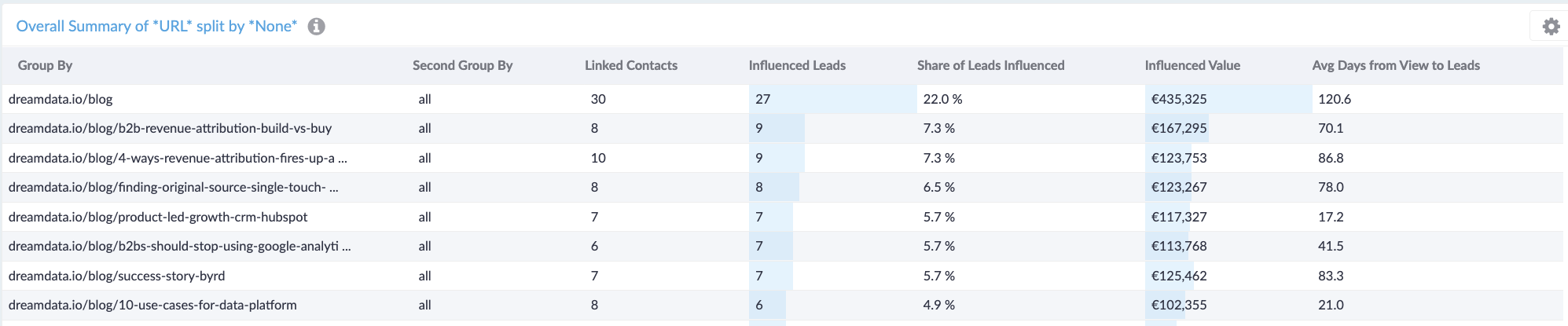
Lastly, the table shows the average number of days between the content was viewed and when the lead was generated. A high number of days indicate that on average there is a long time between MQL readers view the page and when they became an MQL. On the other hand, a low number of days indicate that for readers becoming an MQL does so short time after reading the content.